top of page
I used this function to see how many rows and columns this data set contains
preprocessing: Text

preprocessing: Image
I used this function to see the names of columns in this dataset. Through the names of columns, I am able to have the insight or the initial understandings about this dataset.
preprocessing: Text

preprocessing: Image
I used this function to see the first 5 rows of the dataset to make a quick observation about the values.
preprocessing: Text
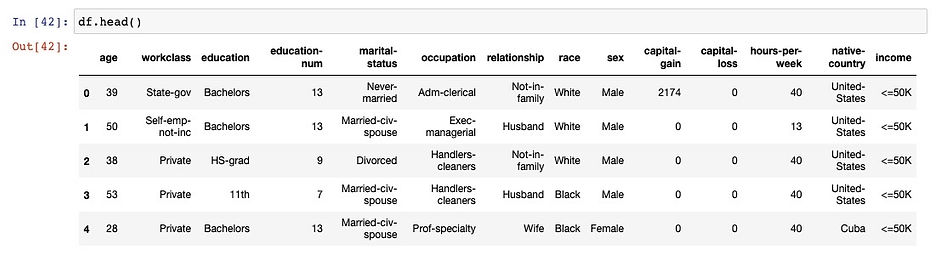
preprocessing: Image
After observing the first 5 rows of this dataset, I noticed that the 'capital-loss' columns contains all 0 values and the 'capital-gain' also contains all 0 values except the first row. I decided to observe the first 50 rows of this dataset to see if these two columns contain all 0 values or not. If these two columns contain all 0 values, I will drop them.
preprocessing: Text

preprocessing: Image
After observing the first 50 rows, I noticed that these two columns contain other values beside 0 value so I can conclude that these 0 values have their own meaning then I will not drop these two columns.
Next, I will observe to see if the data set still has null or missing values.
preprocessing: Text

preprocessing: Image
Fortunately, this dataset does not contain any missing values. Even though this dataset does not contain any missing value, but let check if it contain any weird value. I will randomly pick the 'workclass' column to check.
preprocessing: Text
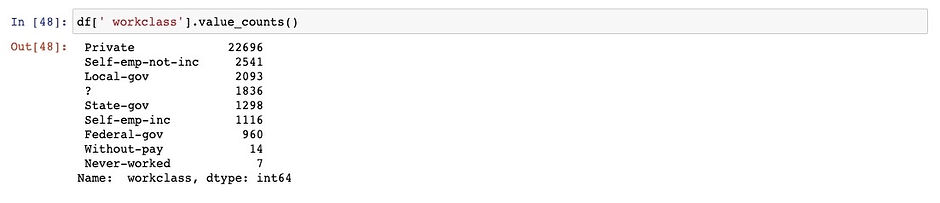
preprocessing: Image
Surprisingly, this 'workclass' column contains a good amount of "?" values, Let pick another column to check if only this 'workclass' column contains '?' values or other columns also contain '?' values. I will pick 'occupation' column to check.
preprocessing: Text

preprocessing: Image
Here I noticed that 'occupation' column also contains these weird '?' values. I will drop all these '?' values at all the columns.
preprocessing: Text

preprocessing: Image
Check again if there are still these '?' values in this dataset.
preprocessing: Text
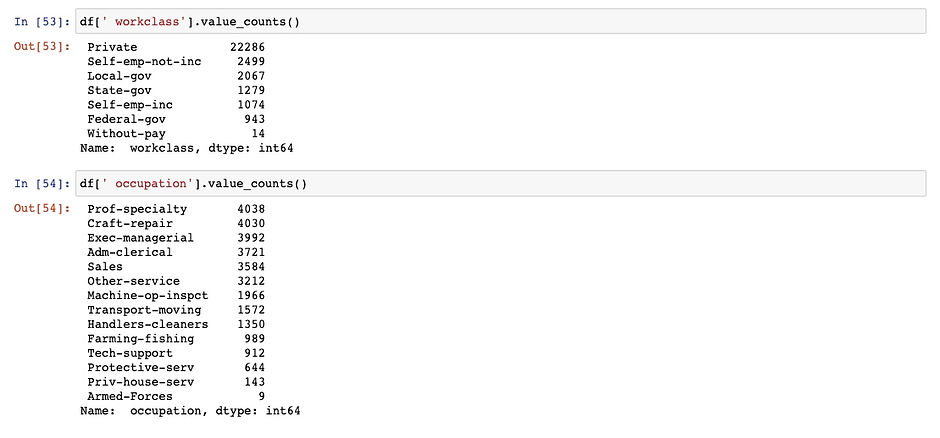
preprocessing: Image
Great! All the wierd '?' values are removed!
Next, we notice that the 'fnlwgt' variable is a weight that is given by a researcher arbitrarily, it will not be neceesary and useful for the purpose of this project and my intended use of this dataset so I will drop this column!
preprocessing: Text
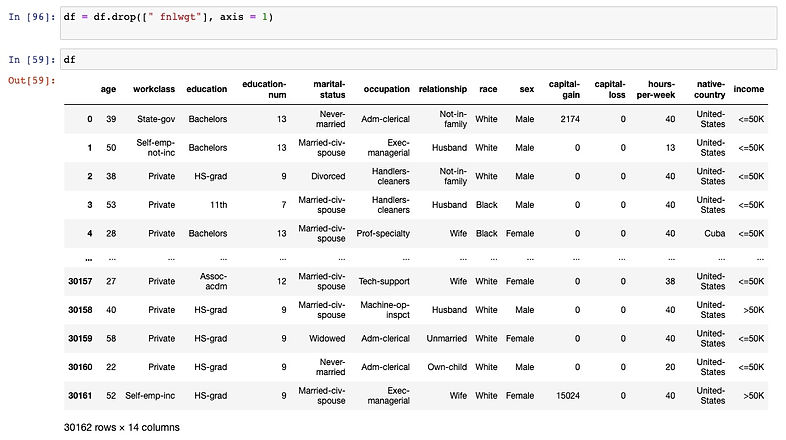
preprocessing: Image
preprocessing: Text
bottom of page