top of page
I will observe to see if the data set still has null or missing values.
preprocessing: Text
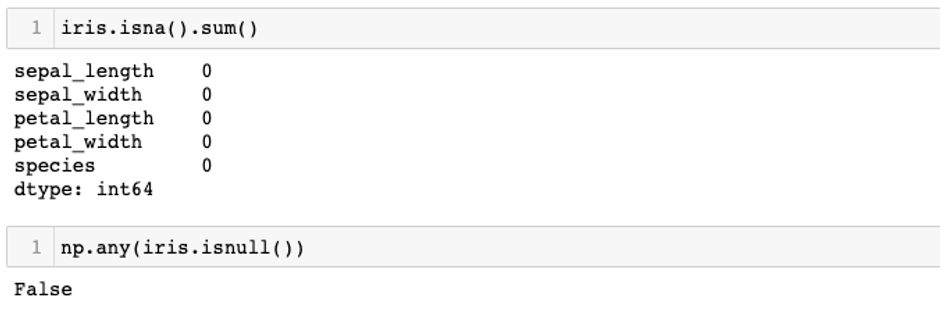
preprocessing: Image
Fortunately, this dataset does not contain any missing values. Therefore, I do not have to drop any row that contains missing values. Moreover, since all the columns in this dataset is necessary for building and training the clustering model so I also will not drop any column.
Notably, since the data type of all the feature columns that I will use to build and train my clustering model are numerical data, which is suitable for my purpose so I also will not transform the data type of any data.
Overall, since this Iris dataset from scikit-learn is a clean, neat and ready to use dataset so I do not have to do much in this preprocessing step.
I will separate the dataset into X containing the features, which are petal_length, petal_width, sepal_length, sepal_width.
preprocessing: Text

preprocessing: Image
T containing the target, which is the different types of specie (setosa, versicolor, virginica).
preprocessing: Text
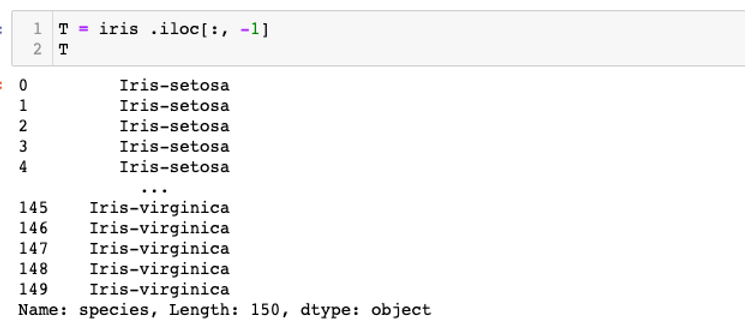
preprocessing: Image
I will use the X dataset to build and training my clustering model and ignore the T dataset that contains the target. However, I will use the T to evaluate if my clustering model perform well on this Iris dataset or not.
preprocessing: Text
preprocessing: Text
bottom of page