Before building any classifier model, we need to scale the data first since some numerical data in this dataset has differnt scales, which is needed to be scaled before moving on.
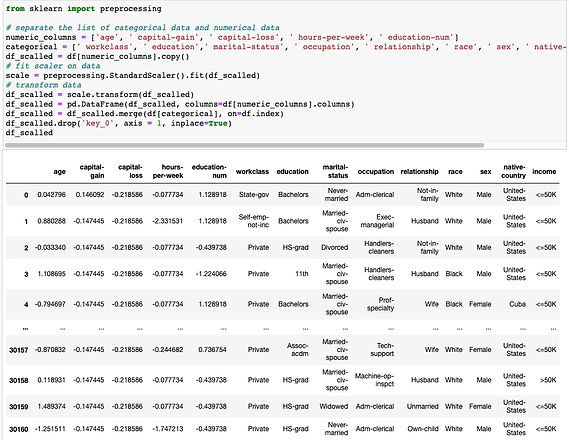
We need to encode our dataset first since this income dataset contains both numerical and categorical data.

We need to create the target dataframe and feature dataframe which is used to train our model.
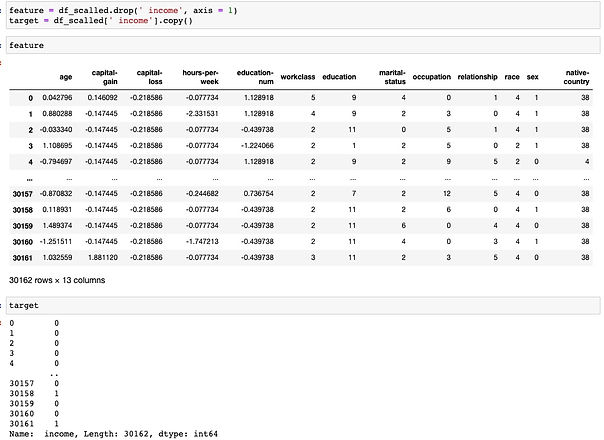
Next, we need to split the data.
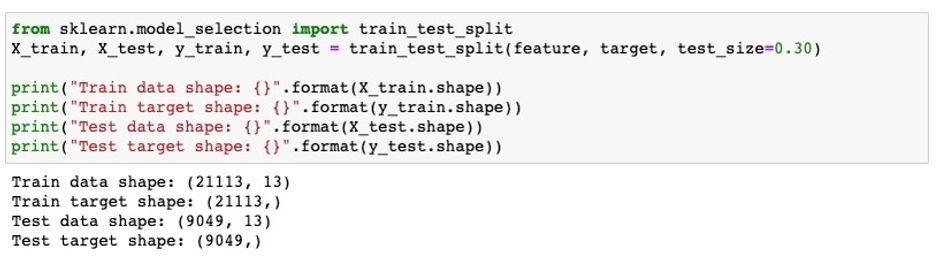
Decision Tree
There are some reasons that made me choose decision tree for this project. First of all, this classifier algorithm is easy to understand and to interpret. Moreover, decision tree is able to handle both numerical and categorical data. Since this income dataset I chose contain both numerical and categorical data so decision tree is definitely the best choice to do prediction on this dataset.
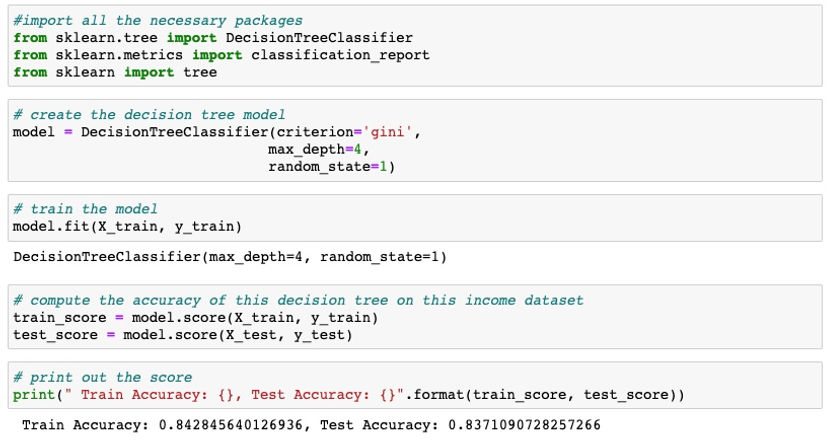
We can see that the test accuracy score of this decision tree classifier on this income dataset is pretty high. In other words, the decision tree classifier did a pretty good job on classifying in this income dataset.
I will plot the tree plot to show how this decision tree classifier works on this income dataset.
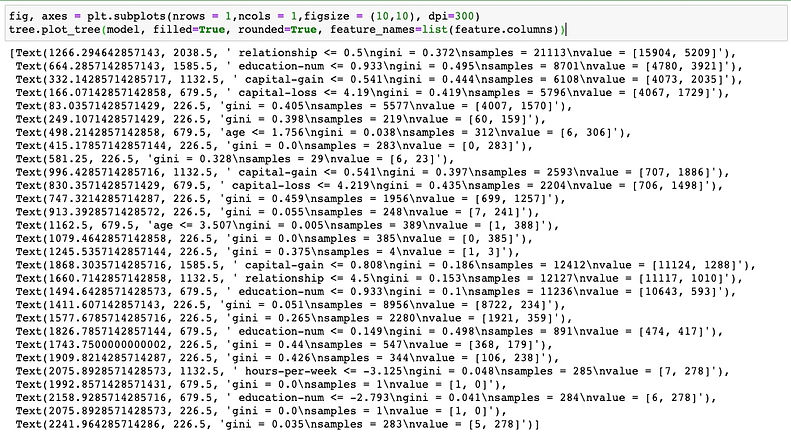
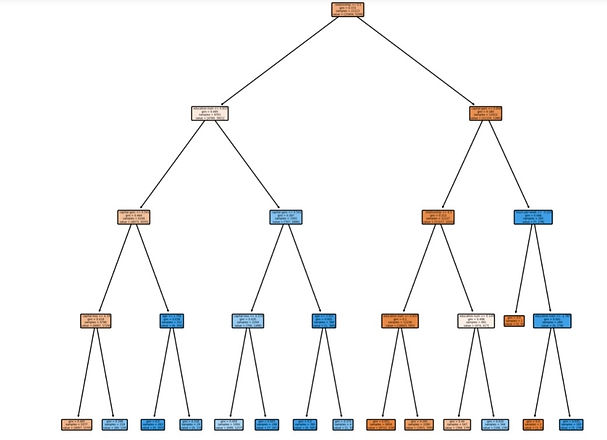
Now, lets compute the train and test prediction of decision tree classifier on this income dataset.

Pipelined SVC
Support Vector Machines (SVM) is widely used in classification objectives, which is totally suitable for the purpose of this project. Support vector machine is highly preferred by many as it produces significant accuracy with less computation power. The reason I chose SVM with pipeline is to make a comparison with decision tree to see which classification algorithm works better on this income dataset.
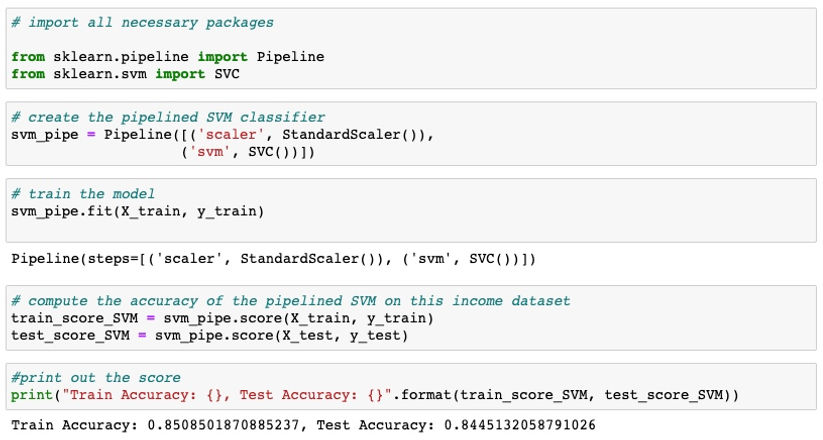
Now, let's compute the train and test prediction of pipelined SVM classifier on this income dataset.
