top of page
The first thing I observed that this dataset contains lots of NaN values and some of the columns contain all the NaN values. I will print out all the columns containing missing values and the number of missing values for each of those column.
ex1 preprocessing: Text

ex1 preprocessing: Image
Looks like the majority of data in 'Alley' column, 'FireplaceQu' column, 'PoolQC' column, 'Fence' column and 'MiscFeature' column contain NaN values so I will go ahead and drop all these columns.
ex1 preprocessing: Text

ex1 preprocessing: Image
For the numerical data containing the less number of missing values, I will replace all the missing values by the median of corresponding column. For the categorical variables containing missing data, I will fill in None to all those missing categorical data.
ex1 preprocessing: Text
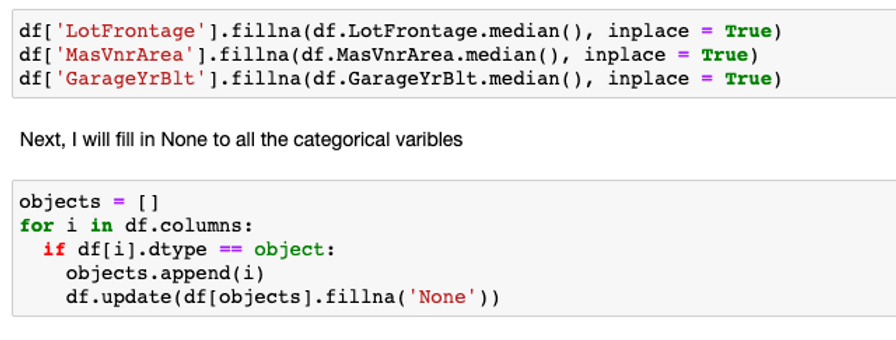
ex1 preprocessing: Image
Now lets check if my dataset still contains any missing value or not.
ex1 preprocessing: Text

ex1 preprocessing: Image
Great! Now my dataset is clean of missing values.
Next, I will make sure that all the variables will be transformed to numerical values in order to build and train regression model on this dataset.
ex1 preprocessing: Text

ex1 preprocessing: Image
Lastly, I will also drop the ID column since this column is not necessary for building and training any model.
ex1 preprocessing: Text
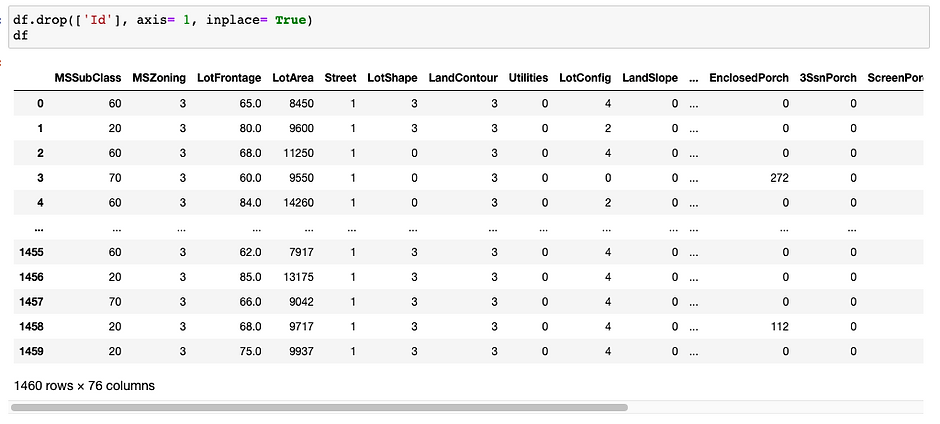
ex1 preprocessing: Image
ex1 preprocessing: Text
bottom of page